

A sincere understanding and empathetic interpretation of another individual’s journey this does not mean the actor’s rendition will be without flaw, but it can encourage a higher degree of understanding for any observer of the method or performance.īrad Pitt, an actor who needs no introduction, once said: “There are no Hallmark cards that define the next chapter, or the value of a history together”. The phenomenal aspect that this method can promote within an actor, performer or at the most impressive level, a human, is a remarkable insight into another person’s life. This is also referred to as the “art of experiencing”.
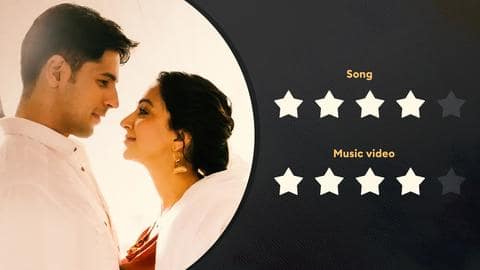
We’ll discuss this dataset in more detail in the next section.There is a technique in acting known as the: “Stanislavski’s system for acting” (created and named after Russian actor and director Konstantin Stanislavski ) which encourages actors to focus on their characters’ inner lives rather than outer expressions.
#Song track separator download
There’s no need to download the dataset, we will provideĬode for obtaining the clips later on in the tutorial. More specifically, we’ll use short clips from this dataset. In this tutorial we’ll be using the MUSDB18 dataset. Separating a specific instrument or voice from the mix, separating into groups such as harmonic versus percussive instruments, or separating a mix into all of More recent datasets typically includeįull duration songs recorded in stereo and provide all isolated stems, allowing us to choose which source separation problem we want to address, whether it’s Separating vocals from the accompaniment without providing access to all isolated stems (instruments) comprising the mix.
#Song track separator full
With which it was trained, so if your goal is to separate stereo audio recorded at 44.1 kHz, ideally you will trained your model on audio in the same format.Īs we can see, earlier datasets were smaller in terms of the number of tracks, sometimes only providing short clips rather than full songs, and often focused on Mixtures in terms of the number of instruments is unlikely to successfully separate dense mixtures.ĭuration: Does the dataset provide full-length songs, or just excerpts (clips) from songs? The former is a richer data source.įormat: Are the tracks provided as stereo audio or mono? What’s the sampling rate? Typically a source separation algorithm will output audio in the same format Instruments: Which ones? How many? A model is unlikely to successfully separate an instrument it hasn’t seen during training. If we want our trained model to generalize well to music of a certain genre, it is important for that genre to be well represented in our training data. Musical genre, while inherently aįuzzy concept, is a reasonable proxy for the types of mixtures we can expect in terms of instrumentation and arrangement, playing styles, and mixing characteristics. Musical content: Our models are unlikely to generalize well to mixtures that are very different from those used during training. But quantity isn’t enough! We need quality and variability too, as captured by Number of tracks: Generally speaking, the more the better. The columns of the table indicate the key characteristics we must consider when choosing or curating a dataset for music source separation: This extended table is based on: SigSep/datasets, and reproduced with permission. Here’s a quick overview of existing datasets for Music Source Separation:
